Single image super-resolution (SR) methods aim at recovering high-resolution (HR) images from given low-resolution (LR) ones. SR Algorithms are mostly learning-based methods that learn a mapping between the LR and HR image spaces (see Fig.1) . Among SR methods present in the literature, there are Super-Resolution Convolutional Neural Networks and Fast Super-Resolution Convolutional Neural Networks, among the most used ones.
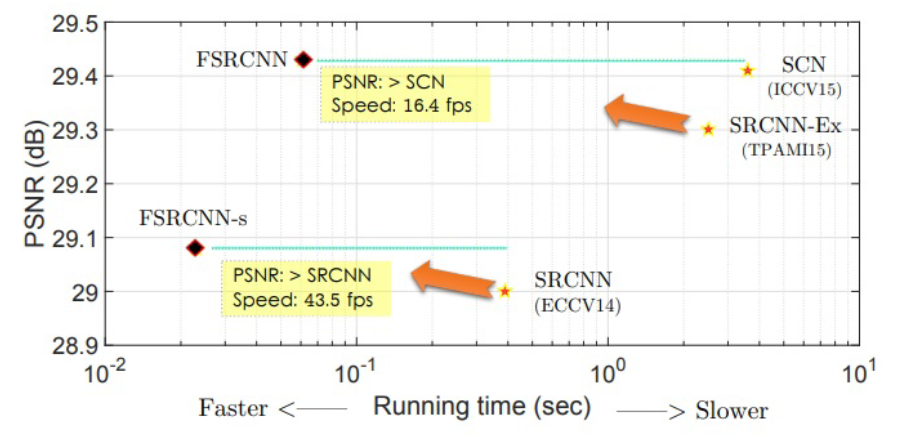
Fig.1. Comparative performance of the SRCNN and FSRCNN super-resolution method.
FSRCNN: A improvement of the SRCNN method, which adopts the original low-resolution image as input. This method is divided into five parts:
Feature Extraction: Bicubic interpolation in previous SRCNN is replaced by 5×5 conv.
Shrinking: 1×1 conv is done to reduce the number of feature maps from d to s where s<<d.
Non-Linear Mapping: Multiple 3×3 layers are to replace a single wide one
Expanding: 1×1 conv is done to increase the number of feature maps from s to d
Deconvolution: 9×9 filters are used to reconstruct the HR image.
The FSRCNN is different from SRCNN mainly in three aspects. First, FSRCNN adopts the original low-resolution image as input without bicubic interpolation. A deconvolution layer is introduced at the end of the network to perform upsampling. Second, the non-linear mapping step in SRCNN is replaced by three steps in FSRCNN: the shrinking, the mapping, and the expanding step. Third, FSRCNN adopts smaller filter sizes and a deeper network structure. These improvements provide FSRCNN with better performance but lower computational cost than SRCNN.
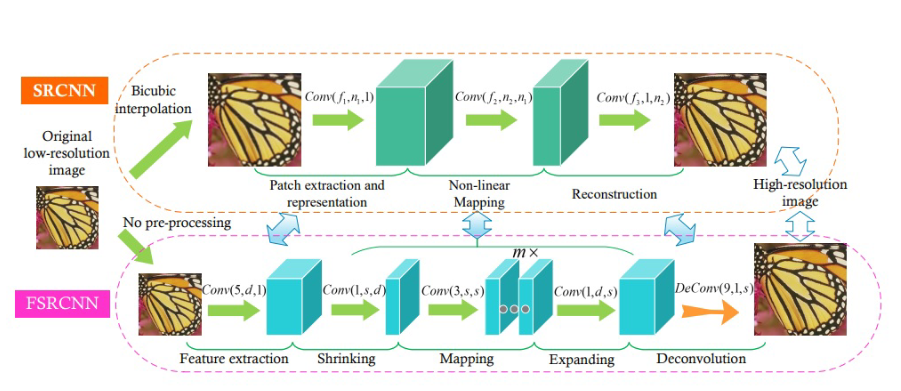
Fig.2. Network structures of the SRCNN and FSRCNN methods.
Structure of FSRCNN
For the implementation of the FSRCNN, we implemented a model with eight layers, where layer 1 is the Feature extraction, layer 2 is, layers 3–6 are denoted to figure 3, layer seven carrier out expanding, and layer 8 performs the deconvolution function. The layers are defined as follows:
Conv. Layer 1 "Feature extraction": 56 filters of size 1 x 5 x 5.Activation function: PReLU. Output: 56 feature maps; parameters: 1 x 5 x 5 x 56 = 1400 weights and 56 biases
Conv. Layer 2 "Shrinking": 12 filters of size 56 x 1 x 1. Activation function: PReLU. Output: 12 feature maps; parameters: 56 x 1 x 1 x 12 = 672 weights and 12 biases
Conv. Layers 3–6 "Mapping": 4 x 12 filters of size 12 x 3 x 3. Activation function: PReLU. Output: HR feature maps; parameters: 4 x 12 x 3 x 3 x 12 = 5184 weights and 48 biases
Conv. Layer 7 "Expanding": 56 filters of size 12 x 1 x 1. Activation function: PReLU.Output: 12 feature maps; parameters: 12 x 1 x 1 x 56 = 672 weights and 56 biases
DeConv Layer 8 "Deconvolution": One filter of size 56 x 9 x 9. Activation function: PReLU. Output: 12 feature maps; parameters: 56 x 9 x 9 x 1 = 4536 weights and 1 bias
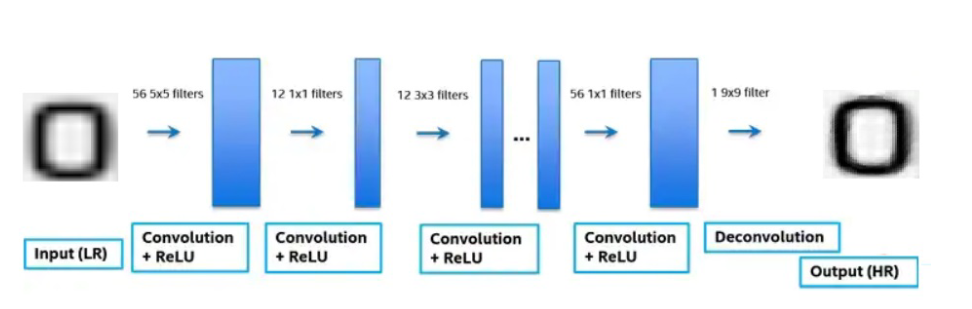
Fig.3.Structure of FSRCNN.
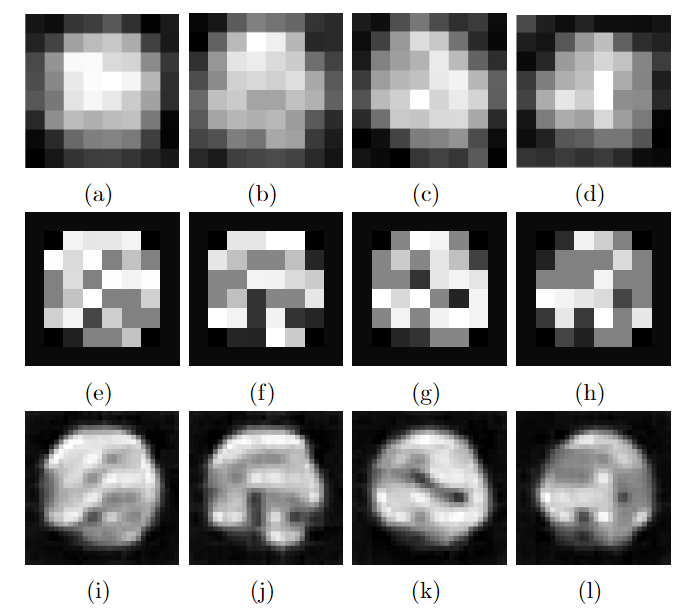
Fig.4: SPI 2D image reconstruction using Batch-OMP algorithm in combination with FSRCNN for the scanning methods: Basic, Hilbert, Zig-Zag, and Spiral. As the test object, a Sphere with 50 mm of diameter placed at 25 cm focal length:(a-d)SPI reconstruction of the 8x8 image using the following scanning methods Basic, Hilbert, Zig-Zag, and Spiral respectively, (e-h) post-processing a method based on the application of a bilateral filter, and(i-l)SPI image obtained after applying the FSRCNN approach.
Comments