Abstract
Recent advancements in vision technology, especially in Single-Pixel Imaging (SPI) cameras, have caught the eye of many in the tech world. This document delves into this cutting-edge imaging method's progress and potential uses. By leveraging advanced reconstruction algorithms, SPI can create images from compressed data collected by just one detector element. This technology's development towards smaller, more integrated forms has allowed it to be embedded in lightweight mobile devices, broadening its application spectrum. Real-time imaging and video recording achievements enable capturing and examining moving scenes. Through innovative hardware and computational strategies, improvements in sensitivity and resolution have been realized. Applying deep learning techniques further advances the imaging process and helps extract valuable insights from the gathered information. Fields as varied as medical imaging, biophotonics, object identification, tracking, remote sensing, observation of the Earth, industrial inspection, and quality control are reaping the benefits of SPI technology. The ongoing progress in SPI camera technology is set to transform numerous sectors, opening up exciting new possibilities for imaging and analysis.
Challenges Faced by Single-Pixel Imaging Technology
In the last ten years, there has been an impressive leap forward in technologies such as autonomous robots, self-driving cars, and drones. Alongside this, there has been remarkable progress in improving vision systems with innovative methods. Various sensors, like LiDAR, RADAR, thermal, and infrared (IR) cameras, have played a crucial role in these advancements. A groundbreaking development in vision technology is the use of the SPI system. This new approach allows sensors to adjust to various wavelengths, from visible light to near-infrared (IR) and even longer wavelengths. The key benefit of this flexibility is its effectiveness under challenging weather conditions like fog, rain, or low visibility. The SPI system maintains its efficiency by merely changing the light source, without reconfiguring the SPI setup. As commonly presented in academic studies, SPI's potential uses go well beyond theoretical concepts. For instance, it can be applied to create an effective obstacle-detection system for vehicles in foggy or rainy weather. Furthermore, when combined with LiDAR technology, SPI can significantly improve the detail and accuracy of scene reconstruction, achieving a more precise and efficient process by tailoring the number of samples according to the SPI principle.
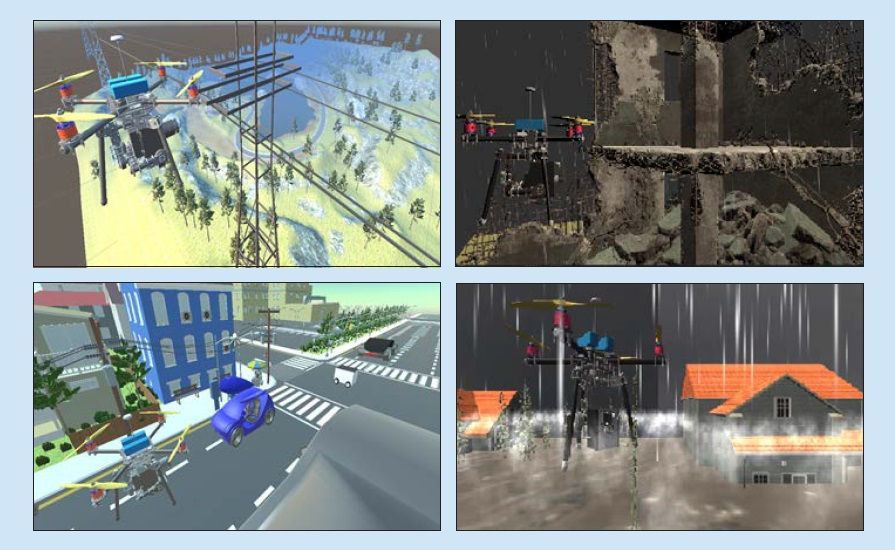
Fig.1 presents a compelling illustration of the possible future uses of single-pixel camera infrared technology, highlighting its improved image-capturing abilities in a range of difficult lighting and scattering scenarios. Such progress is particularly beneficial in intricate areas such as urban landscapes or dense forests.
Integrating Deep Learning Models into SPI Technology
Single Pixel Imaging (SPI) technology represents a significant leap forward in image capture, utilizing a single-pixel detector alongside structured illumination patterns to achieve innovative results. This technique has broadened the horizons for object detection, segmentation, tracking, and depth mapping applications. Enhancements from recent developments in deep learning models have significantly improved SPI's image reconstruction and analysis capabilities. A key innovation in this space is the Single-Pixel Object Detection (SPOD) method, which employs deep learning algorithms to detect and pinpoint objects within a scene through SPI measurements alone. This method is particularly beneficial when traditional imaging struggles, such as in low light or with limited hardware. Another deep learning application within SPI technology is image segmentation. By training neural networks with segmented images reconstructed from single-pixel data, it's feasible to delineate precise object boundaries, leading to superior-quality segmented images. This advancement aids in better object recognition and analysis across various sectors, including medical imaging and autonomous vehicles.

Fig.2. New era in imaging as Deep Learning Models seamlessly merge with SPI Technology.
Deep learning's impact extends to object tracking through single-pixel imaging as well. Utilizing convolutional neural networks (CNNs) to recognize object motion patterns from SPI data enables tracking algorithms to accurately monitor objects across dynamic environments, overcoming obstacles like occlusions or clutter. This opens up new possibilities for sophisticated tracking in surveillance, robotics, and augmented reality. Depth mapping benefits similarly, with deep learning models trained on extensive datasets of single-pixel depth measurements and accurate ground truth depth maps, facilitating the creation of precise depth maps from sparse data. This is crucial for 3D reconstruction, virtual reality, and autonomous navigation. Integrating deep learning with SPI also explores innovative avenues beyond conventional imaging. Combining SPI with Neural Radiance Fields (NeRF), for instance, allows for the reconstruction of detailed 3D human poses, objects, and 4D spatial-temporal data from limited measurements, finding uses in virtual try-on, computer graphics, and telepresence technologies. Moreover, merging hyperspectral imaging and high-speed video with SPI enables the capturing of multispectral information in dynamic scenes. Employing deep learning for data fusion enhances the analysis and comprehension of complex environments and is useful in environmental monitoring and remote sensing.
Deep learning models further elevate SPI quality through techniques like Deep Image Prior (DIP) and diffusion models, which refine and clarify low-resolution or noisy single-pixel images. These approaches leverage learned priors or diffusion processes to improve image restoration and sharpening, delivering higher fidelity in SPI applications. Overall, the fusion of deep learning models with SPI technology marks a series of breakthroughs in the field. From enhancing object detection, segmentation, and tracking to depth mapping and image quality improvement, deep learning algorithms facilitate more precise and efficient image reconstruction and analysis. These advancements not only broaden the applicability of SPI across various domains but also set the stage for ongoing innovation in imaging technology.
BibTeX
@ARTICLE{10472984,author={Quero, Carlos Osorio and Durini, Daniel and de Jesús Rangel-Magdaleno, José and Martinez-Carranza, José and Ramos-Garcia, Rubén},journal={IEEE Instrumentation & Measurement Magazine}, title={Emerging Vision Technology: SPI Camera an Overview}, year={2024},volume={27},number={2},pages={38-47},keywords={Deep learning;Industries;Visualization;Surveillance;Robotvisionsystems;Transfor ms;Cameras},doi={10.1109/MIM.2024.10472984}}
Comments