In this article, we propose a method to be used for the reconstruction of single-pixel near-infrared (SPI-NIR) low-resolution 2D images using active illumination with a peak wavelength of 1550 nm that is based on Batch Orthogonal Matching (Batch-OMP) processing algorithms and a region definition in
the projection sequence of Hadamard illumination patterns using the genetic algorithm(GA). Different methods to generate Hadamard pattern sequences have been reported, mostly based on switching the illumination sequence on and off to improve the reconstructed image quality, thereby increasing the Structural Similarity Index Measure (SSIM) level and reducing the processing time. These methods are efficient for image sizes of>64x64virtual pixels, but for lower resolutions with small coherence areas Ach, the SNR level of the reconstructed image is very low, which makes other methods, such as those using the Zig-Zag or Hilbert filling curves for the scanning path, an option for the reconstruction of SPI-NIR low-resolution images. Because in the present application, we deal with low-resolution (size image 8 x 8 virtual pixels) SPI-NIR images, we present a solution to improve the obtained image quality (aiming at PSNR>10dBand SSIM>0.5) that is based on the use of a specific scanning path and a combination of a genetic algorithm to define the switching sequences of the Hadamard patterns, using Batch-OMP algorithm for image reconstruction in the processing time range between 20 and 35 ms.

Fig.1. Schematic diagram of Hadamard pattern generation using GA: (a) population with Hadamard parent patterns is generated to illuminate the object, (b) after the cost function measurement, these patterns are ranked using the matrix Hp, (c) each new pattern is created through changes in patterns using a mutation operation of the values, (d) the mutated offspring patterns replace the parent generation patterns. These steps are repeated in every generation.
Hadamard genetic algorithm (GA) for region-projection
To define the projection areas Ap, we start by dividing all the Hadamard N x N projection patterns required (for our application, the maximum required number of patterns is 64) into groups of N / 4, each containing a number of N / 16 patterns. These groups will be defined as projection areas and represented as a matrix Hp defined by Eq. (1). The matrix Hp is optimized to increase the quality of the reconstructed image while maintaining the processing time required <30ms, using between 20 and 80% of used Hadamard patterns. As an optimization strategy, a genetic algorithm method GA (see Fig.1) can be applied, in which the elements of the Hp matrix are encoded to form a binary vector (forming the GA initial population vector), where the ”1” elements define the groups without a change throughout the Hadamard sequence of patterns, and ”0” elements represent the groups of matrix elements that change the sequence of Hadamard patterns being sequentially switched ”on” and ”off.”
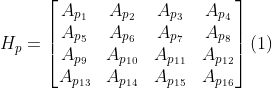
Definition of the most optimum projection of Hadamard patterns using illumination regions defined by the GA algorithm
To determine the most optimal sequence of Hadamard patterns to be projected to obtain the highest possible quality of the reconstructed images, as defined by the application of figures of merit PSNR>10dBand SSIM>0.5, it is necessary to define which projection sequences are necessary to be inverted to comply with our goal. To do this, GA was used to determine the most optimal ”on” and ”off” conditions of the different Hadamard matrix elements throughout the different pattern sequences in an evolutionary way. We used our data-set formed with images of spherical and square objects, using n x n pixel image sizes for the test.
Initially, we defined a Hadamard projection sequence of 64 patterns divided into 16 groups (see Fig. 2b) containing four elements of the projection sequence input element These elements were used to form the vector Vp that contains the matrix elements positions encoded in a binary form, and its dimensions are of 1 x 16. For the GA-based evaluation process, it was necessary to define an initial population of N = 8 divided into six parents. This initial population is an 8 x 16-pixel matrix containing random data. A fitness function was defined to evaluate the projection sequences, which will receive the Vp vector containing the positions of the different Hadamard matrix elements throughout the sequence of generated patterns corresponding to the population to be evaluated. We used the Zig-Zag, Hilbert, and Spiral scanning sequences to reconstruct a comparative study's single-pixel image of interest. (a) (b)

Fig 2: Generation of Ap sequence areas using GA algorithm: (a) Hadamard projection sequence of 64 patterns divided into 16 groups containing four elements of the projection sequence for the Ap projection area. (b) GA algorithm that evaluates the different switching on-off sequences in the Hadamard projection, using the vector Vp with binary positions defined for the different groups.
Comments