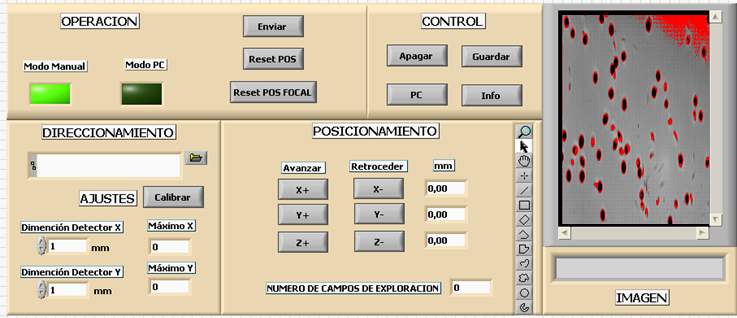
Technical development to interpret etched nuclear tracks induced by single-charged particles or by complex interactions in plastic detectors exposed at accelerators or at GCR during space missions at Low Earth Orbit has received much attention. Frequently, large numbers of tracks are to be analyzed, often several thousand frames are under scrutiny for an individual track detector. Hence automatic processing is required. We show an alternative, new technique to analyze etched tracks by a commercially available product: IMAQ™ Vision Builder6, made by National Instruments. The data acquisition setup is the usual one: the image is collected by a CCD camera and sent to a data acquisition board type IMAQ™ PCI/PXI™-1409 (National Instruments). Then the image is analyzed with a purpose-built algorithm utilizing the IMAQ™ Vision Builder6 software, which includes a set of functions, such as image enhancement, filtration, binary image conversion, pattern recognition, providing frequency histograms for selected track parameters. To evaluate the technique employed with this newly available system, we present some results from a track etch detector evaluation algorithm developed by Pálfalvi et al. at the Atomic Energy Research Institute (Budapest).
23rd International Conference on Nuclear Tracks in Solids, Beijing (China), 11-15 September 2006