Physics-Informed Neural Networks (PINNs) have recently gained attention as an effective approach for tackling complex inverse problems in image processing, especially in image denoising. This paper introduces an innovative framework that utilizes a range of neural network architectures—including ResUNet, UNet, U2Net, and Res2UNet—to implement denoising strategies grounded in nonlinear partial differential equations (PDEs). The proposed methods employ PDEs such as the heat equation, diffusion processes, multiphase mixture and phase change (MPMC) models, and Zhichang Guo's technique, embedding physical laws within the learning process to enhance denoising robustness and accuracy. We demonstrate that these models can be trained to effectively reduce noise while preserving critical image features, using a blend of data-driven methods and physical constraints. Our experiments show that integrating PDEs leads to superior denoising performance compared to traditional techniques. The models were evaluated on multiple datasets, with metrics like Peak Signal-to-Noise Ratio (PSNR) and Structural Similarity Index Measure (SSIM) indicating significant improvements in image quality. These results highlight the potential of using PINNs with nonlinear PDEs for advanced image-denoising tasks, paving the way for future research at the intersection of deep learning and physics-based modeling in image processing.
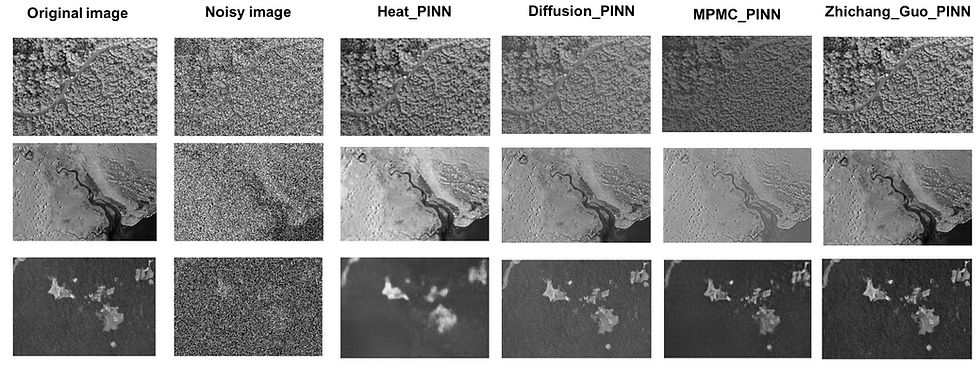