- Carlos Osorio
- 2 abr 2024
- 4 Min. de lectura
Actualizado: 19 abr 2024
Abstract
Capturing 3D details of human pose and body shape from just one monocular image poses a significant challenge in computer vision. Traditional approaches rely on RGB images, which are limited by changes in lighting and obstructions. However, advances in imaging technology have led to novel methods like single-pixel imaging (SPI), which overcome these obstacles. SPI, especially in the near-infrared (NIR) spectrum, excels at detecting 3D human poses. This wavelength can go through clothing and is less affected by lighting changes than visible light, offering a dependable way to capture accurate body shape and pose information, even in challenging environments. In this research, we investigate using an SPI camera operating in the NIR spectrum with time-of-flight (TOF) technology at 850 and 1550 nm wavelengths. This setup is designed to identify humans in low-light conditions. We employ the vision transformers (ViT) model to recognize and extract human features, integrating them into a 3D body model called SMPL-X through deep learning-based 3D body shape regression. To test the effectiveness of NIR-SPI for 3D image reconstruction, we created a lab environment that mimics night conditions, allowing us to explore the potential of NIR-SPI as a vision sensor in outdoor night settings. By analyzing the data from this experiment, we aim to showcase NIR-SPI's capabilities as a powerful tool for nighttime human detection and for capturing precise 3D human body pose and shape.
Human modeling
Using parametric human models, such as SMPL-X, allows for a concise representation of human shapes by utilizing shape and pose parameters to encode variations [6]. The SMPL-X model offers various advantages:
It disentangles the human shape and pose, allowing for independent analysis and control of each shape.
It avoids modeling rugged and twisted shapes directly, which can pose difficulties for neural network-based methods, by utilizing a skinning process to model deformation and
It is differentiable and can be easily integrated with neural networks. For this research, we used SMPL-X as the underlying representation for modeling 3D humans.
Proposed Method
The process used to obtain the 3D human model from NIR-SPI. It involves several steps that use different computer vision techniques to reconstruct a 3D human pose from a single low-resolution image. Here is a detailed explanation of each step:
Take a single-pixel low-resolution image. This step involves capturing an image of a human. The image contrast is adjusted to extract the basic shape of the person, and then the background is removed using U2Net. This deep-learning model can accurately segment the foreground and background of an image. Thus, an image segmentation technique isolates the person from the background to obtain the image’s silhouette. This image only shows the person's outline without any details of the surface or texture.
Applied over the silhouette image, ViT can identify four human poses: lying, bending, sitting, and standing. Once the pose is identified, it can be used to generate a 3D human pose using the VIBE method, a deep learning model that can estimate the 3D pose of a human from a single image or video.
Finally, we can reconstruct the human body shape and pose in 3D space. As discussed above, this can be done using a tool such as SMPL-X.
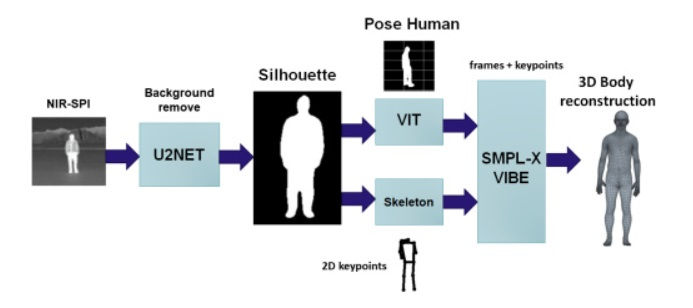
Fig.1. Overview of the proposed network architecture, which takes NIR single-pixel imaging input and outputs 3D body reconstruction based on SMPL-X shape and pose parameters. The entire network consists of three main modules: (i) NIR-SPI-based image acquisition. (ii) Feature extraction using deep learning: The NIR-SPI image extracts the background to obtain the silhouette. (iii) 3D pose estimation using a regression-based approach: The silhouette image is used to obtain the gait features (shape estimation), which are then used to pose the human using ViT and skeleton joint features. These features are used to pre-define the pose SMPL-X model; from the pre-defined parameters (pose 𝜃, shape 𝛽 and camera s, R, T), the SMPL-X model is fed to the off-the-shelf SMPL-X model to obtain the reconstructed 3D human mesh.

Fig.2. Capture human pose imaging at a distance of 1 m: (a) Capture NIR-SPI imaging of human pose standing, sitting, and bending, (b) silhouette image, and (c) 3D human pose regression based on SMPL-X model.
The proposed methods to obtain a 3D human model from NIRSPI imaging, for human poses such as lying, bending, sitting, and standing. The best accuracy was achieved in the sitting position, with an accuracy of around 91%, as shown in the V2V and MPJPE errors. The results demonstrate the effectiveness of the proposed approach, with limitations in hand positioning due to the low contrast of the NIR-SPI image. However, the level position of the core person detection shows an accurate estimation of the 3D pose of the person through qualitative and quantitative evaluations. These findings highlight the potential of the proposed approach for 3D human modeling from a single low-resolution image.
In comparison, the presented SMPL-X model captures the body, face, and hands jointly, and the SMPL-X approach fits the model to a single NIR-SPI image and 2D joint detections. The results of this work demonstrate the expressivity of SMPL-X in capturing bodies, hands, and faces from NIR-SPI images. However, we observed that the bending and lying pose presented the highest V2V and MPJPE error levels, indicating limitations in the pose parameters θ. Therefore, it is recommended that a compensation model be implemented in future applications. Future work may involve the development of a dataset of in-the-wild SMPL-X fits and the direct regression of SMPL-X parameters from NIR-SPI images.
BibTeX
@article{OsorioQuero:24, author = {Carlos Osorio Quero and Daniel Durini and Jose Rangel-Magdaleno and Jose Martinez-Carranza and Ruben Ramos-Garcia}, journal = {J. Opt. Soc. Am. A},keywords = {Image metrics; Imaging techniques; Machine vision; Single pixel imaging; Three dimensional imaging; Three dimensional reconstruction}, number = {3}, pages = {414--423}, publisher = {Optica Publishing Group}, title = {Enhancing 3D human pose estimation with NIR single-pixel imaging and time-of-flight technology: a deep learning approach}, volume = {41}, month = {Mar}, year = {2024}, url = {https://opg.optica.org/josaa/abstract.cfm?URI=josaa-41-3-414},doi = {10.1364/JOSAA.499933},}
Enhancing 3D human pose estimation with NIR single-pixel imaging and time-of-flight technology: a deep learning approach. Journal of the Optical Society of America A. Vol. 41, Issue 3, pp. 414-423 (2024) https://doi.org/10.1364/JOSAA.499933