top of page
Ph.D. Electronic Engineer
Research Blog
Buscar
18 abr 20232 Min. de lectura
Actualizado: 6 nov 2024
Phase retrieval is an important problem in many fields, including image processing, optics, and computer vision. It refers to the process of recovering the phase of a signal from its magnitude. In recent years, deep learning has emerged as a powerful tool for image processing tasks, including phase retrieval. In this article, we will explore the concept of phase retrieval in image processing and discuss the use of untrained deep learning models for phase retrieval.
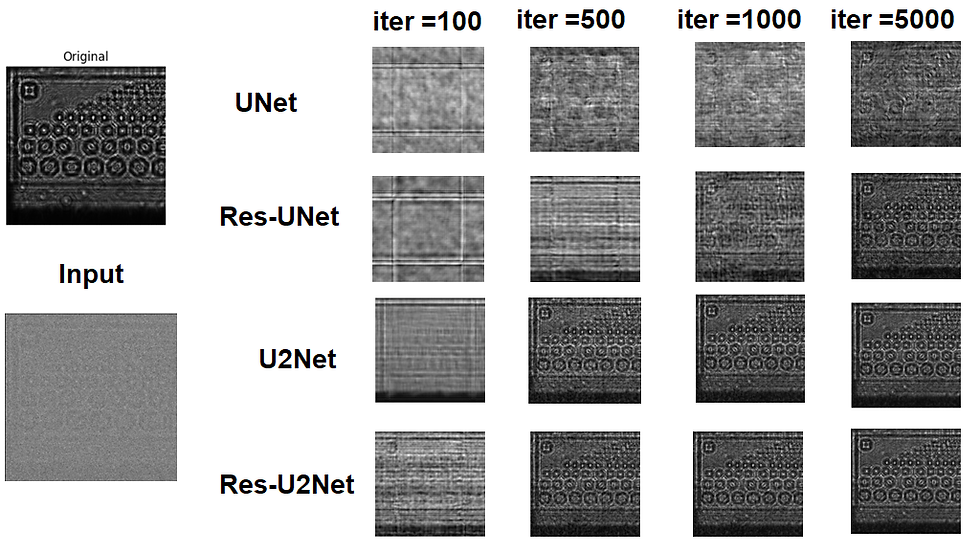
Phase Retrieval in Image Processing
In image processing, phase retrieval refers to the process of reconstructing an image from its magnitude. The magnitude of an image is the absolute value of its Fourier transform, while the phase is the angle of the Fourier transform. Phase retrieval is necessary in many applications where only the magnitude of the image can be measured. For example, in X-ray crystallography, the intensity of the X-ray diffraction pattern can be measured, but the phase information is lost. Similarly, in microscopy, the phase information of an image is often lost due to the use of lenses. Phase retrieval can be approached using various algorithms, including the Gerchberg-Saxton algorithm, the Fienup algorithm, and the alternating projection algorithm. These algorithms typically require prior knowledge of the phase or some initial estimate of the phase. However, in some cases, this prior knowledge may not be available or may be inaccurate, making the phase retrieval problem challenging.
Untrained Deep Learning for Phase Retrieval
Deep learning has been successfully applied to various image processing tasks, including image restoration, denoising, and segmentation. Recently, deep learning has also been applied to phase retrieval. One approach is to use untrained deep learning models for phase retrieval. These models are trained on a large dataset of random phase images and their magnitudes. During inference, the model is presented with the magnitude of an image and is expected to output the phase. The use of untrained deep learning models for phase retrieval has several advantages. First, it eliminates the need for prior knowledge of the phase or some initial estimate of the phase. Second, it can handle noise and other distortions in the input magnitude. Third, it can be trained on a large dataset of random phase images, making it applicable to a wide range of problems. One popular untrained deep learning model for phase retrieval is the U-Net. The U-Net is a convolutional neural network that has been widely used for image segmentation tasks. However, it can also be used for phase retrieval by treating the magnitude of an image as the input and the phase as the output. The U-Net architecture consists of a contracting path, which encodes the input image into a latent space, and an expanding path, which decodes the latent space into the output phase.
Phase retrieval is an important problem in image processing, and deep learning has emerged as a powerful tool for addressing this problem. Untrained deep learning models, such as the U-Net, have been shown to be effective for phase retrieval, eliminating the need for prior knowledge of the phase or some initial estimate of the phase. The use of untrained deep learning models for phase retrieval has the potential to revolutionize the field of image processing, making it applicable to a wide range of problems.
3 abr 20231 Min. de lectura
SMPLX Human is a cutting-edge human model generated using single-pixel imaging technology. This model represents a significant leap forward in the field of computer graphics and machine learning, as it allows for the creation of highly realistic and accurate 3D models of human subjects. Single-pixel imaging is a technique that involves using a single light source and a detector to capture information about an object's appearance. This information is then processed using algorithms to generate a 3D model of the object. The SMPLX Human model takes this process one step further by applying machine learning to the data obtained from single-pixel imaging.
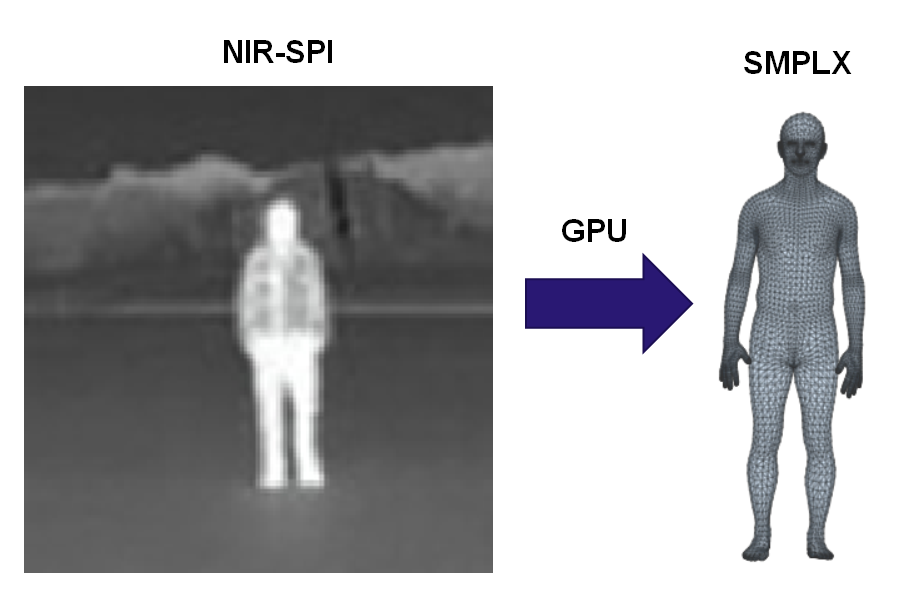
To generate the SMPLX Human model, researchers first captured single-pixel images of a diverse range of human subjects. These images were then processed using machine learning algorithms to extract detailed information about the subjects' bodies, including their body shape, pose, and clothing. This information was then used to generate highly realistic 3D models of each subject.
The SMPLX Human model is highly versatile and can be used for a wide range of applications, including virtual reality, computer graphics, and robotics. Its high level of accuracy and realism makes it an invaluable tool for industries such as film and gaming, where lifelike human characters are in high demand.
The generation of the SMPLX Human model using single-pixel imaging technology and machine learning algorithms represents a significant advancement in the field of computer graphics and machine learning. Its highly accurate and realistic 3D models of human subjects have numerous applications and are sure to have a significant impact on a wide range of industries in the years to come.
bottom of page